Improving the hit rate and bestseller management are essential elements of retail assortment planning and allocation. Learn how to fully understand your bestsellers and how they affect your hit rate to make optimal use of your resources.
Brick and mortar stores were hit hard by Covid-19, especially fashion retailers. And although street footfall is on the rise again, it remains far below 2019 numbers in most European high streets and shopping centres. Kaufinger Street in Munich for example, one of the top 5 German shopping destinations, reports an incredible increase of 344% versus last year but is still 30% short of the YTD (Year-to-Date) footfall in 2019. And let’s not forget that 2019 and the preceding years already saw shrinking traffic across most European shopping destinations.
While retail rental fees have also been under pressure, profitably operating a fashion retail store remains a challenge in most European high streets and shopping centres. Hence, making the best use of available retail space by focusing design and product development resources on the categories with the highest incremental bestseller potential becomes one of the most important strategies in retail assortment planning and allocation.
My last article was about identifying your true bestsellers by evaluating the hit rate of different categories and price points. Here I focus on understanding why a product becomes a bestseller, applying these insights to future bestsellers, and improving your hit rate in the process.
1. Which Products Earn the Title Bestseller?
Companies use different key performance indicators (KPI) and perspectives to identify their bestsellers. While quite straight forward for a retailer with only one point of sale (POS), it becomes challenging for retailers that operate multiple stores in different location categories and of different shapes and sizes.
Between using overall sales volume (total number of pieces sold per item), sell-through rates (share of pieces sold without mark-downs on total number of pieces purchased), stock turn (COGS, i.e. Cost of Goods Sold divided by average inventory) or a combination thereof, to identify your true bestsellers is a question of perspective.
Whatever metric you use, it needs to relate to the type and number of POS where the product is stocked. A product that you stocked in 100 POS will naturally rank higher on your bestseller list than a second product that was available only in 10 POS. A product that is in stock with five pieces per size in your bigger stores will sell better and faster than the same product offered in smaller stores with only one or two pieces per size.
We use the Average Rate of Sale (ARoS) to identify bestsellers and calculate the hit rate of a certain assortment category. For a more in-depth exploration please have a look at this article about the hit rate and bestseller management using ARoS as a KPI.
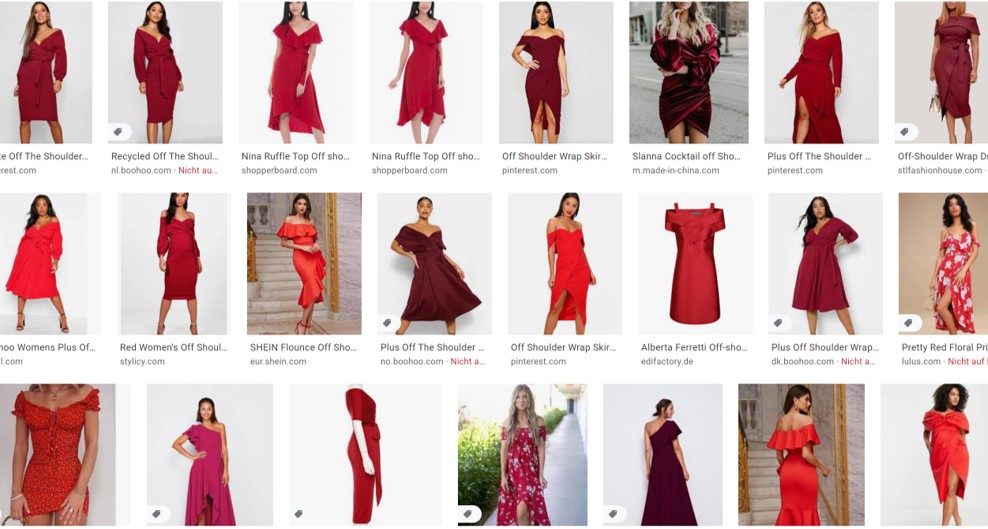
Red-Off-Shoulder-Drape-Dress Variety (Photo: Google)
2. Hit Rate in Brick and Mortar Retail Assortment Planning
The Hit Rate tells you how many styles or articles of your entire collection or of a certain category are bestsellers (or at least good sellers) and what share of your assortment they account for. This is based on your individual benchmark definition of a bestseller, which can differ by category, assortment planning module, lifecycle, price point and the average ARoS as well as its range from minimum to maximum ARoS.
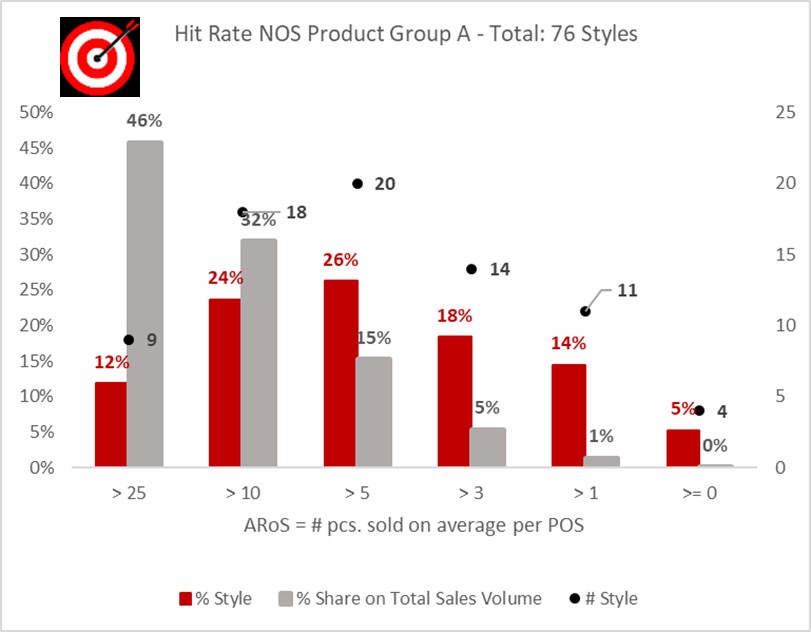
Hit Rate category A – NOS (Graphic: Heike Blank)
In this real-life example we defined bestsellers as items that sell more than 25 times per POS over their lifecycle of 6 months. In comparison, ‘good’ sellers sell between 10 and 25 times, while ‘OK’ sellers sell between 5 and 10 times. For a fast fashion retailer like ZARA, however, a bestseller might be an item that sells 25 times per week.
The bestseller hit rate in this example is 12%. How to read this: 12% of all styles (9 out of 76) sold more than 25 times on average per POS in which they were available. These 9 bestsellers generated 46% of the total sales volume and, with a lifecycle of 6 months (26 weeks), only these 9 styles sold at least once per week per store. The good seller hit rate is 24%. There are 18 styles that sell between 10 and 25 times, generating 32% of total sales volume. Imagine the incremental sales potential if you were able to turn just half of these good sellers into bestsellers.
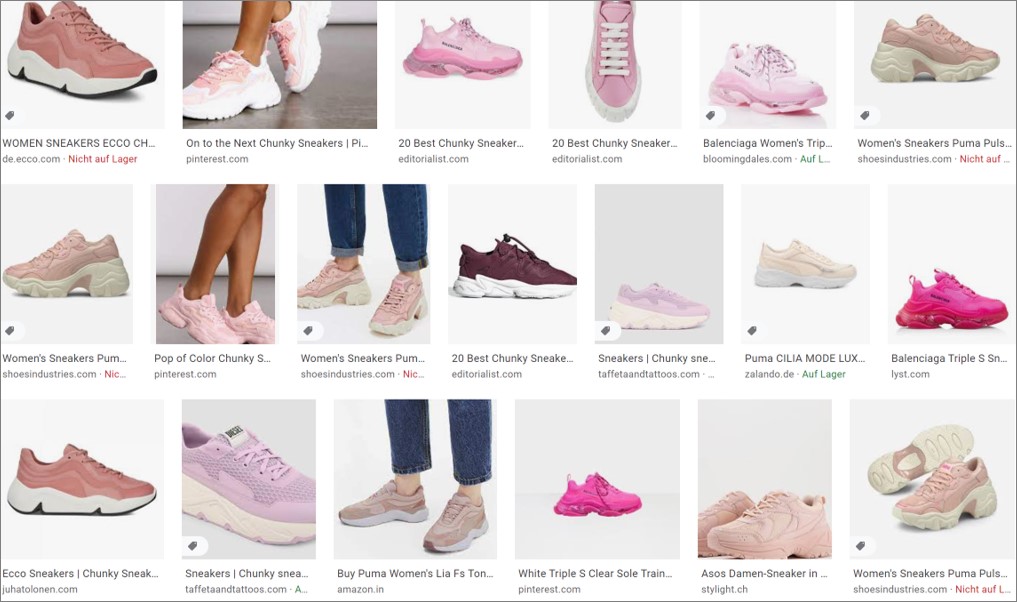
Which of these pink chunky sneakers with a tonal sole will become a bestseller and why? (Photo: Google)
3. What Makes a Product a Bestseller
Truly understanding why one product sells so much better than another in the same category is crucial to unlocking incremental sales potential. Unfortunately, many designers and product managers are too busy with their daily business to spend enough time exploring precisely what the deciding success factor of each individual bestseller is. There are many contenders:
Hard factors can be identified and understood with quantitative analytics:
- Retail assortment planning module: It might be the module it belongs to, i.e. our bestseller is the perfect match for another item that is only available in that particular module.
- Retail locations and how you cluster them: Orange items are always a good guess for bestsellers in the Netherlands but nowhere else (unless it becomes a global trend colour, which happens every 5-10 years).
- Marketing: The item might belong to a promotion campaign that exposes it to a wider public compared to other products that are only exposed to consumers visiting the store.
- Visual Merchandising: The item might be part of your window decoration or a featured item in store.
- Size range and split: Sufficient availability of best-selling sizes is often a precondition for a bestseller.
- Price point: The product may be cheaper than a comparable product, or consumers may compare it to a higher priced item that they consider overpriced.
- Weather: Whether you like it or not, weather is an essential factor in consumers’ purchasing decisions.
- Competition: The product may be perfect, but a close competitor carries a comparable item that is even better or cheaper.
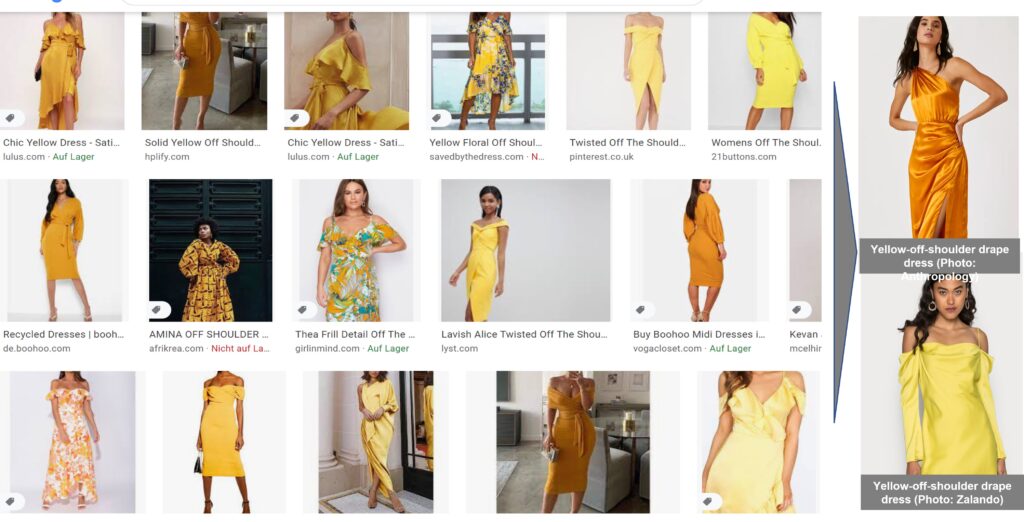
The art of understanding bestseller soft factors (Graphic: Heike Blank)
Soft factors depend on the evaluation of individual preferences and taste:
- Colour
- Pattern
- Material
- Stylistic features such as fit, drape, detailing, styling, branding or its absence
- Functionality: An additional function that products of this category don’t normally have may be doing the trick – things like a water repellent finish, reversibility, a detachable hood, multiple styling options, the number and shape of pockets, etc.
4. How to Identify and Evaluate Soft Factors to Improve your Hit Rate?
It’s quite straight forward to read and interpret the hard factors using an up-to-date MM&A (merchandise management and allocation) tool or ERP system. But it gets more complex and much less predictable when it comes to individual preferences and taste.
Predictive analytics using artificial intelligence, machine learning, deep learning, image processing technology, virtual and augmented reality or natural language processing technology try to provide answers.
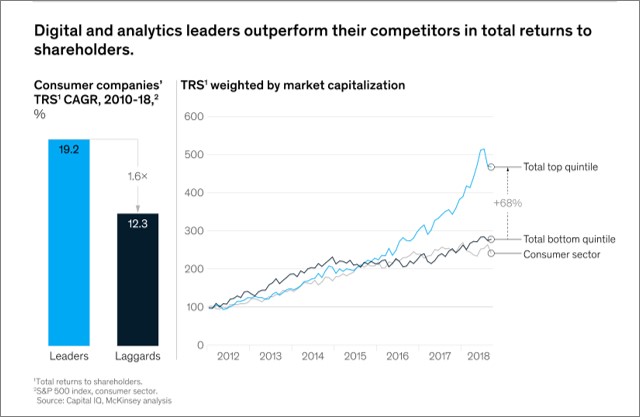
Digital leaders outperform competition (Graphic: McKinsey)
A recent report by Mckinsey & Company shows that consumer goods companies using predictive analytics and leveraging them across the entire company outperform their competition by 68%. And great tools are already available: Edited.com may still be the best one for fashion retailers, but others like 7T, Heuritech or Stylesage are catching up.
5. The Human Factor to Understanding Bestseller Mechanisms
Don’t get me wrong, I certainly like numbers and the stories they can tell us. I am fascinated by the innovations and the progress predictive analytics are achieving in the fashion industry. But any algorithm used to analyse and predict consumer behaviour and bestsellers is only as good as the humans who train the machine, the input it is given to process and the people who have toread, understand and interpret the results.
Predictive analytics depend on historical data. Many smart people are working on teaching their algorithms what gut feelings mean and how to use them for better retail assortment planning. And this is where it gets tricky. Especially in the fashion industry, where many soft factors not only change from customer to customer but also from day to day or hour to hour for the same customer.
Analysing soft factors strongly depends on the many features available to assess and evaluate an item. Such features can be visual and thus analysed by the means of image processing technologies. They can also be the verbal expressions used to describe them. These verbal expressions are called ‘tags’ and they can be as numerous and complex as our language allows.
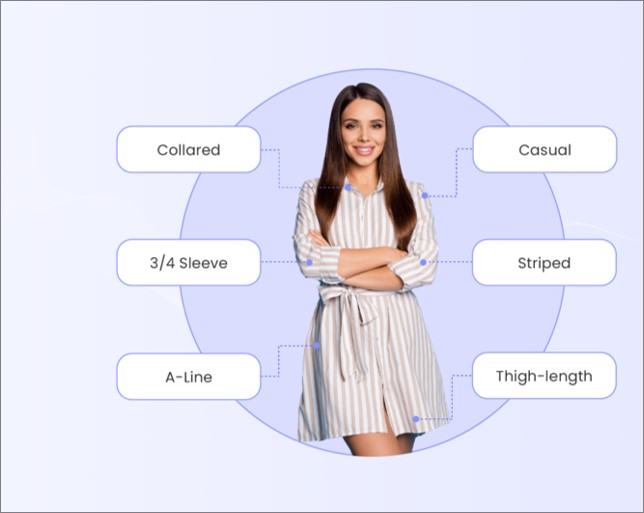
Smart tagging (Graphic: vue.ai)
6. Smart Tagging, Not Only for Predictive Analytics
Structuring, enriching and maintaining your product master data is key to understanding what makes a product a bestseller and how to use these insights to increase your hit rate. And this is where it often goes wrong in the design and product development process:
Creatives lack the time or capacity to extensively describe and tag the product. Administrators, on the other hand, lack the designers’ insights to fill the gaps and feed the machines with detailed information. Over all, not enough time is spent on figuring out what the deciding factor(s) may have been. Add to this the time passed between designing a product and analysing its sell-through, sometimes 6 or 12 months, and an unstructured process, and you can see why using bestseller evaluation to improve hit rates is more difficult than it sounds.
The problem with tagging fashion items is the sheer number of options available to describe and cluster a product. Lets have a look at a few examples:
Colour and pattern tags
Identifying whether a garment is blue or yellow sounds easy. But you also need to understand what shade of blue or yellow it is and why this particular shade worked well this season but not in the previous one.
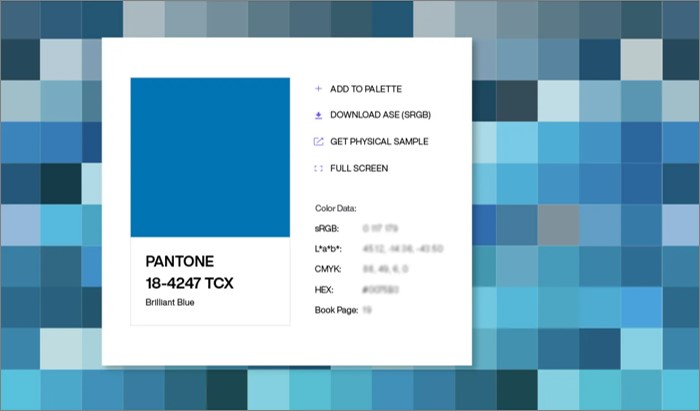
Shades of blue: (Graphic: Pantone)
For monochrome garments this is easy enough; image processing works quite well to identify colour shades. It gets really tricky when trying to tag multicoloured patterns.
Material and drape tags
Material composition is an integral part of any product master data set. But tagging gets more difficult for material blends where you need to decide down to what level of detail you want to structure your tags. Do you use one tag for all wool-polyamide blends regardless of proportions, or do you use one tag for each blend such as 60/40, 70/30, 80/20 and so on?
Even once your material tags are structured appropriately, they won’t tell you anything about the drape of said materials in combination with a particular fit for a particular body type and size. And even if you tagged all these intricate combinations, you still won’t know which consumers enjoyed it and whether they would consider buying a successor product with the same tag combination again.
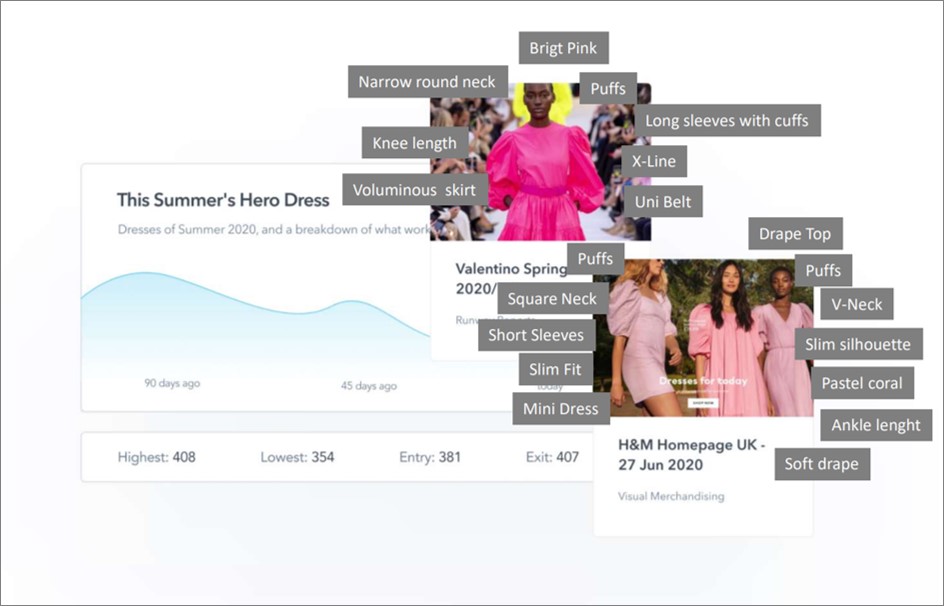
Tagging soft factors for bestseller understanding (Graphic: Heike Blank; Photos edited)
Add the diversity of language and preferences used to describe garments for different target audiences, it becomes clear just how complex the task of fully understanding bestsellers to improve your hit rate is. AI-based tagging tools may be the solution. They facilitate the entire tagging process from defining the attribute structure and content up to maintaining and constantly improving it, and interfacing it with your ERP system and other analytics tools you may use. Examples include Folio3, vue, Ximilar or pixyle.
But despite predictive analytics based on smart tagging, you will still need to spend ample time reviewing the analysed data: Compare it to previous experiences, consider and evaluate the context, and finally make a data-driven guess of what might sell best next season. And that’s precisely where most companies just don’t focus enough, the most common excuse being a lack of time.
Understanding the true success factors of an individual bestseller and using these insights to improve your hit rate will not only boost the top and bottom line of your P&L. It will also save time by eliminating slow sellers and cut the work involved in their design and development, placing them on retail shelves, and eventually getting rid of them. In our earlier example, 37% of all styles sold fewer than 5 times in 6 months. That’s fewer than once every 5 weeks! Imagine the time gained by eliminating just half of these products. And imagine the profit gain you could achieve by increasing your hit rate by the means of a deeper understanding of your bestseller mechanisms!
About the Author:
Heike Blank has worked for big organisations such as VF Europe and s.Oliver but also for niche brands such as Ecko Unltd. and Zoo York in top executive positions. Her extensive experience with opening and managing own retail, partner stores, concessions and shop-in-shops in 23 countries in Europe, the Middle East and Asia make her an expert in expansion. Get in touch with her here and read more of her work here.